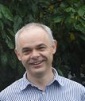
Dirk Mallants
Commonwealth Scientific and Industrial Research Organisation, Australia
Title: Constraining groundwater flow model uncertainty: Workflow and application to deep low-permeable formations
Biography
Biography: Dirk Mallants
Abstract
Large-scale groundwater flow model predictions are generally highly uncertain due to poorly constrained hydrogeologic properties, boundary conditions and geologic layers. The present study demonstrates a generic workflow to iteratively constrain prediction uncertainty by obtaining improved estimates of the most sensitive model components and incorporating them in updated simulations. A case study was developed in which an initially poorly characterized low-permeable formation, a so-called aquitard, was subject to improved characterization resulting in better constrained estimates of hydrogeologic properties. This study used an existing large-scale groundwater flow model in the Gunnedah Basin, New South Wales, Australia as the basis for the quantification of prediction uncertainty. Predictions generated by the flow model included the magnitude and timing of maximum drawdown in a confined aquifer separated from a stressed groundwater system by a sequence of deep aquitards. A global sensitivity analysis revealed the vertical hydraulic conductivity (KV) as one of the most sensitive groundwater system parameters, which was subsequently subject to improved characterization. Porosity-permeability relationships obtained on core samples were combined with downhole neutron-density logs to generate high-resolution vertical KV profiles. These were then up-scaled to the scale of the aquitard formation using analytical and numerical methods. Monte Carlo sampling from hydrogeologic parameter prior distributions was used to estimate the uncertainty of groundwater model predictions before and after improving the characterization of aquitard KV values. Independent estimates of aquitard-scale KV were derived from a 1D transport model interpretation of a profile of natural tracer. Monte Carlo simulations using a spatially uniform aquitard KV model did not considerably change groundwater model predictions. The groundwater model predictions were best constrained when the spatial correlation structure of KV was explicitly accounted for in the Monte Carlo simulations. The workflow allows for an iterative updating of those uncertain parameters to which the model is most sensitive.